Content-based Neighbor Models for Cold Start in Recommender Systems
ACM RecSys Challenge 2017
Winner
Content-based Neighbor Models for Cold Start in Recommender Systems
Abstract
In this paper we address the cold start problem in recommender system by providing a standardized framework to benchmark cold start models.
Cold start remains a prominent problem in recommender systems. While rich content information is often available for both users and items few existing models can fully exploit it for personalization. Slow progress in this area can be partially attributed to the lack of publicly available benchmarks to validate and compare models. This year’s ACM Recommender Systems Challenge’17 aimed to address this gap by providing a standardized framework to benchmark cold start models. The challenge organizer XING released a large scaled data collection of user-job interactions from their career oriented social network. Unlike other competitions, here the participating teams were evaluated in two phases – offline and online. Models were first evaluated on the held-out offline test set. Top models were then A/B tested in the online phase where new target users and items were released daily and recommendations were pushed into XING’s live production system. In this paper we present our approach to this challenge, we used a combination of content and neighbor-based models winning both offline and online phases. Our model produced the most consistent online performance wining four of the five online weeks, and showed excellent generalization in the live A/B setting.
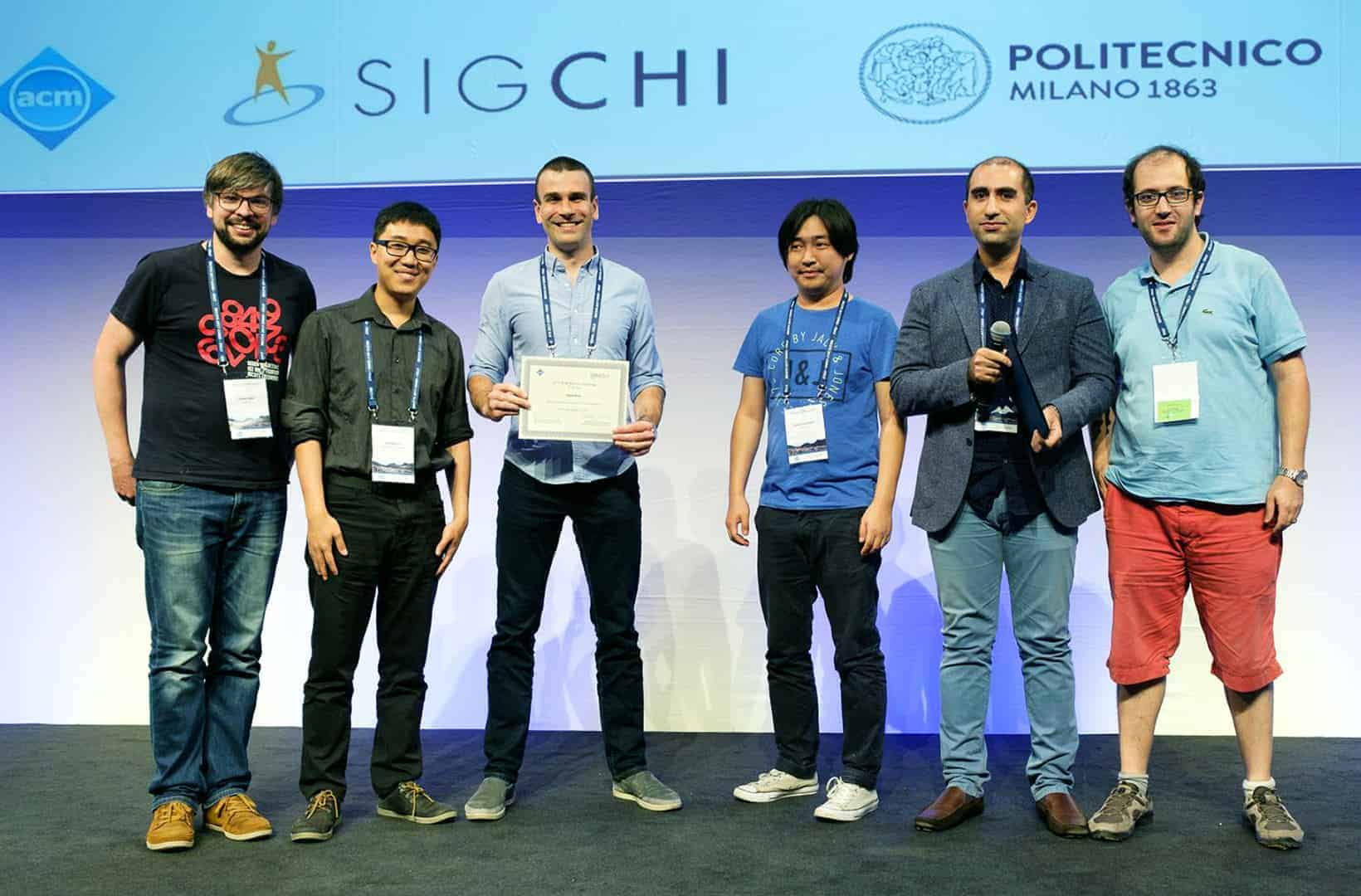