Machine learning research with positive real-world impact.
We develop and deploy industry-leading machine learning systems. Our initiatives have the power to uplift large populations, while advancing the field of artificial intelligence.
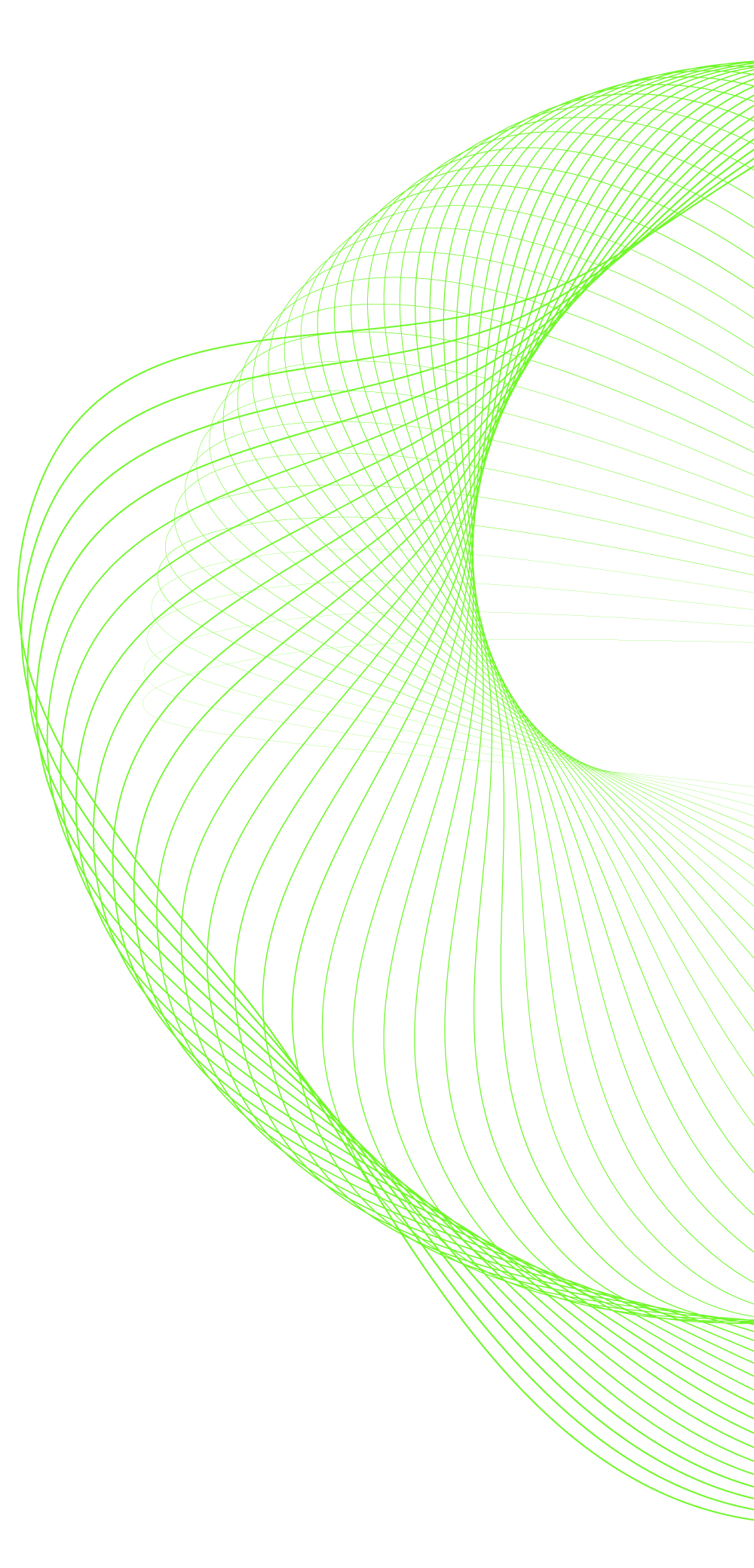
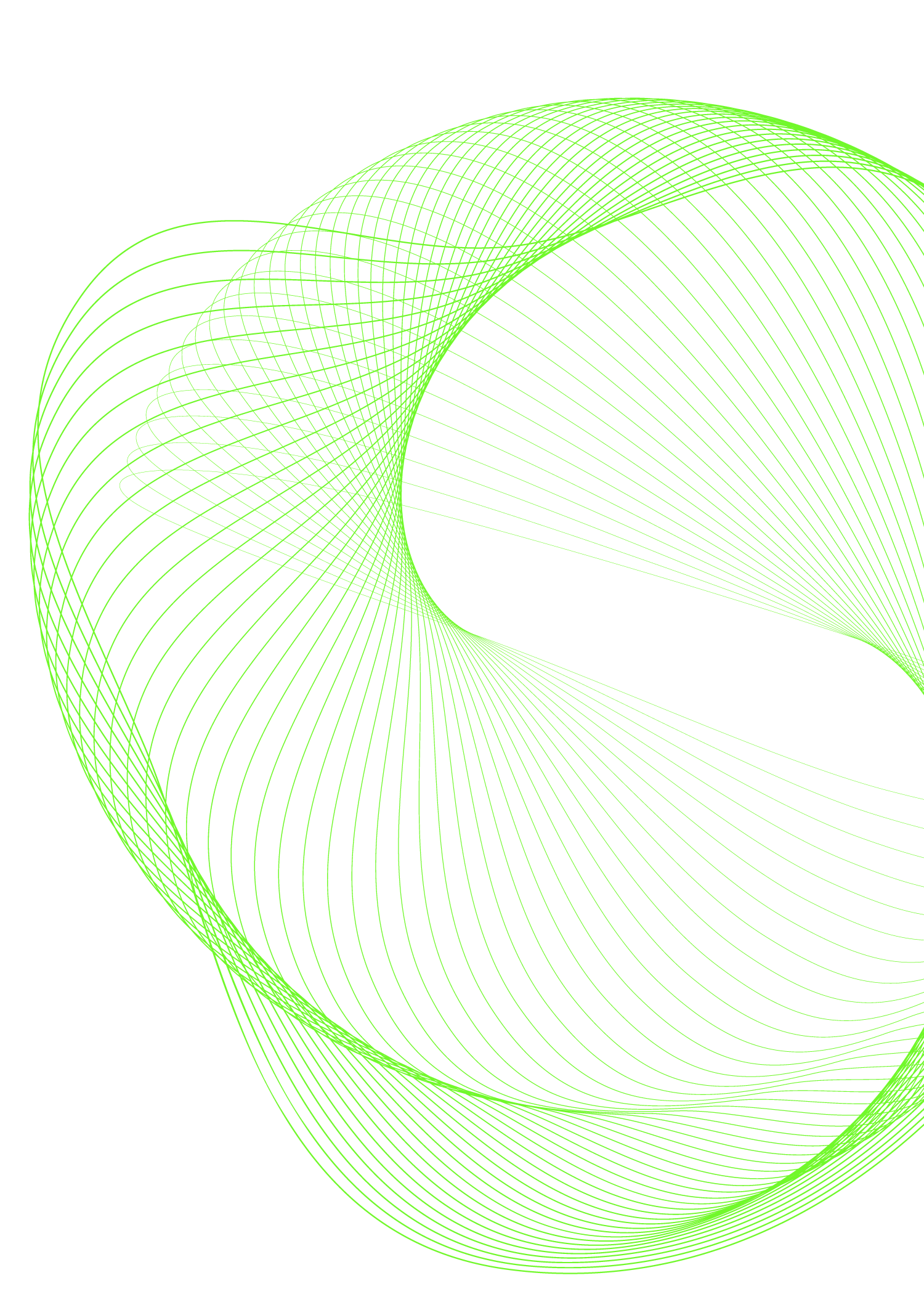
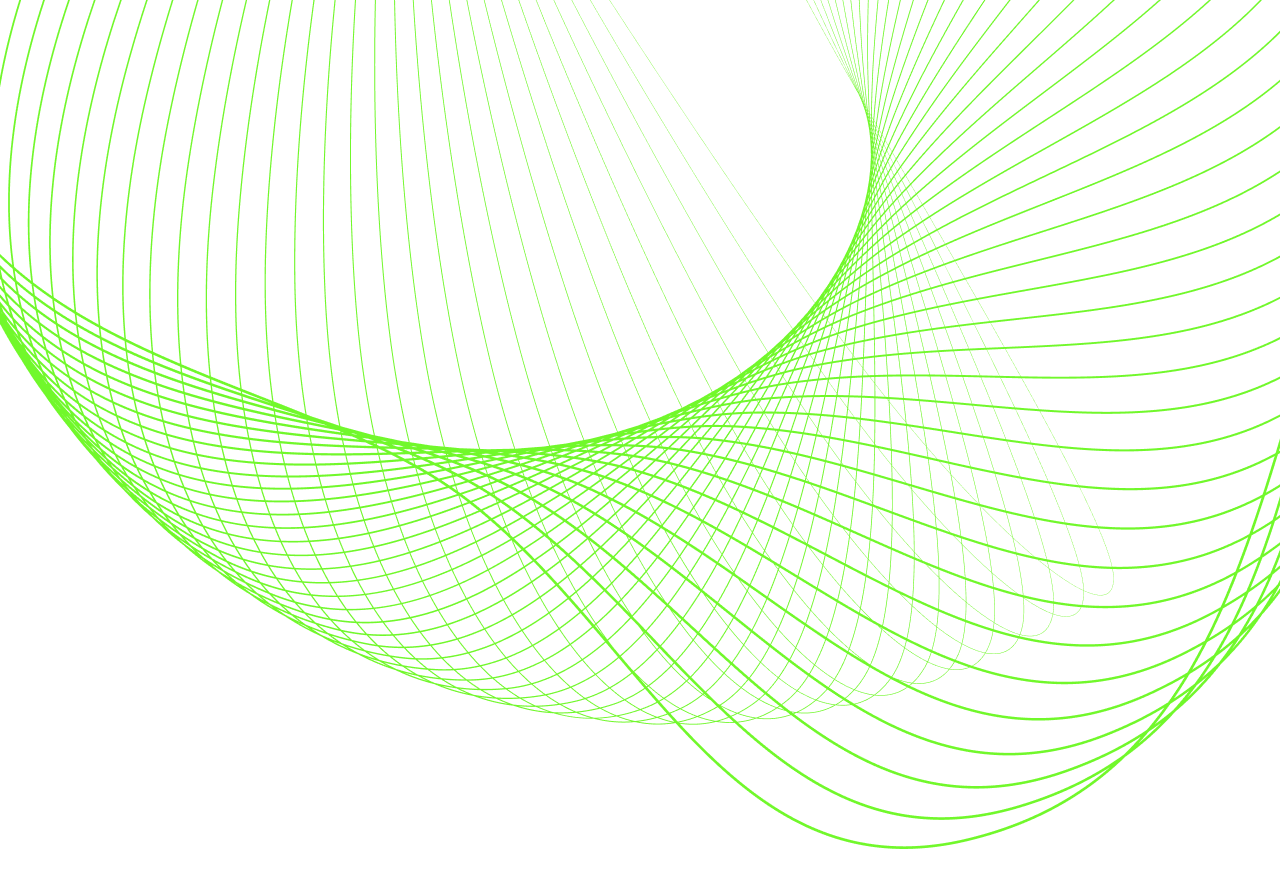
Improving Trustworthiness of AI through Conformal Prediction
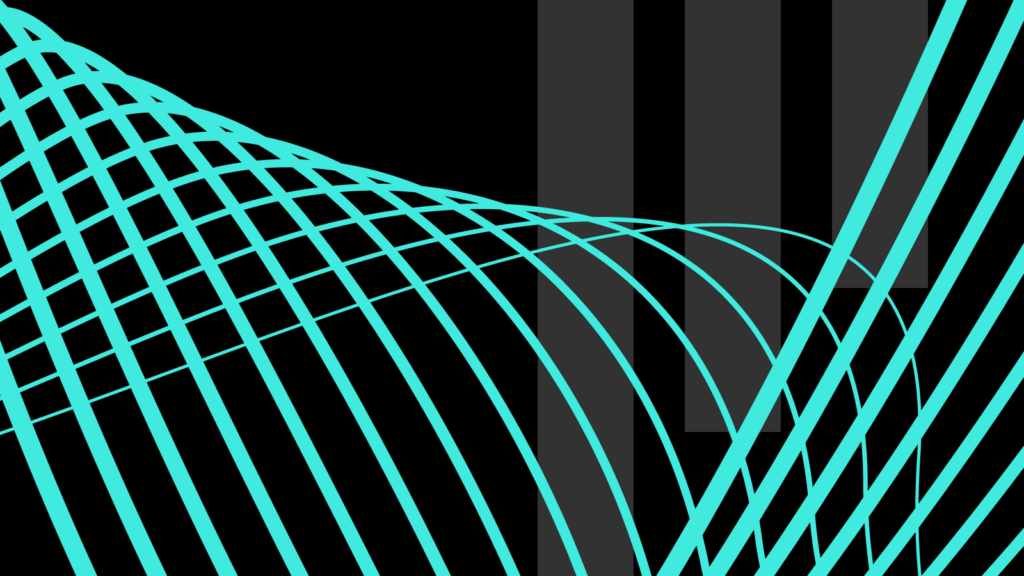
Person to person communication is incredibly nuanced. Beyond the words we choose, our tone, body language, and facial expressions all communicate a great amount of information about how we feel...
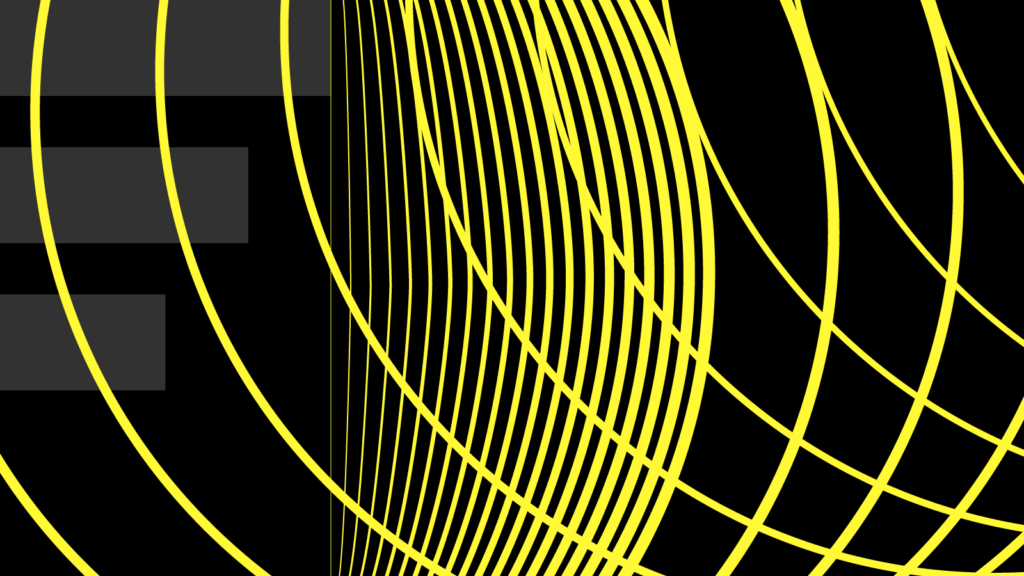
Engineering, /
Regression Testing when Deploying ML Pipelines
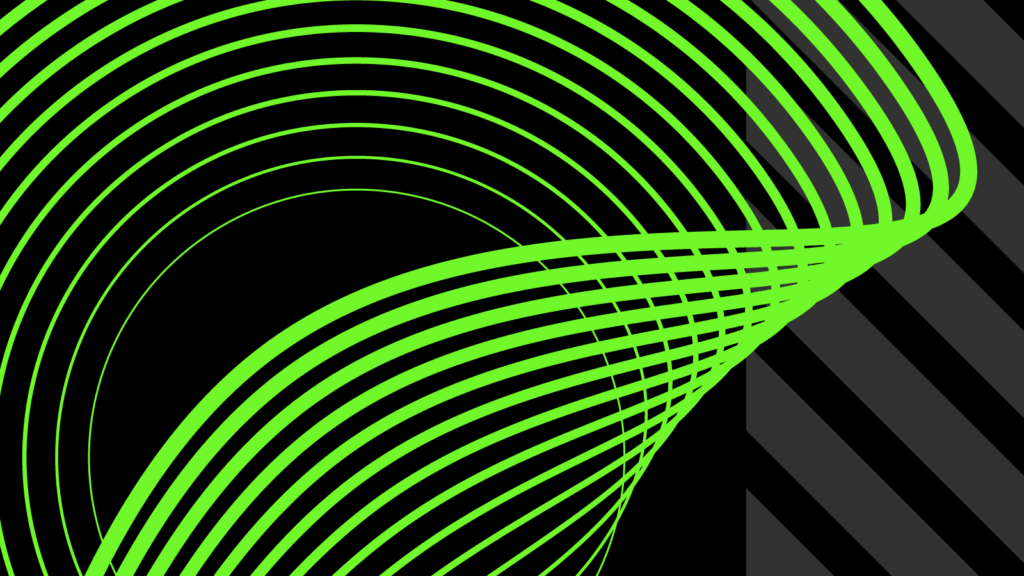
Research, /
Data-Efficient Multimodal Fusion on a Single GPU
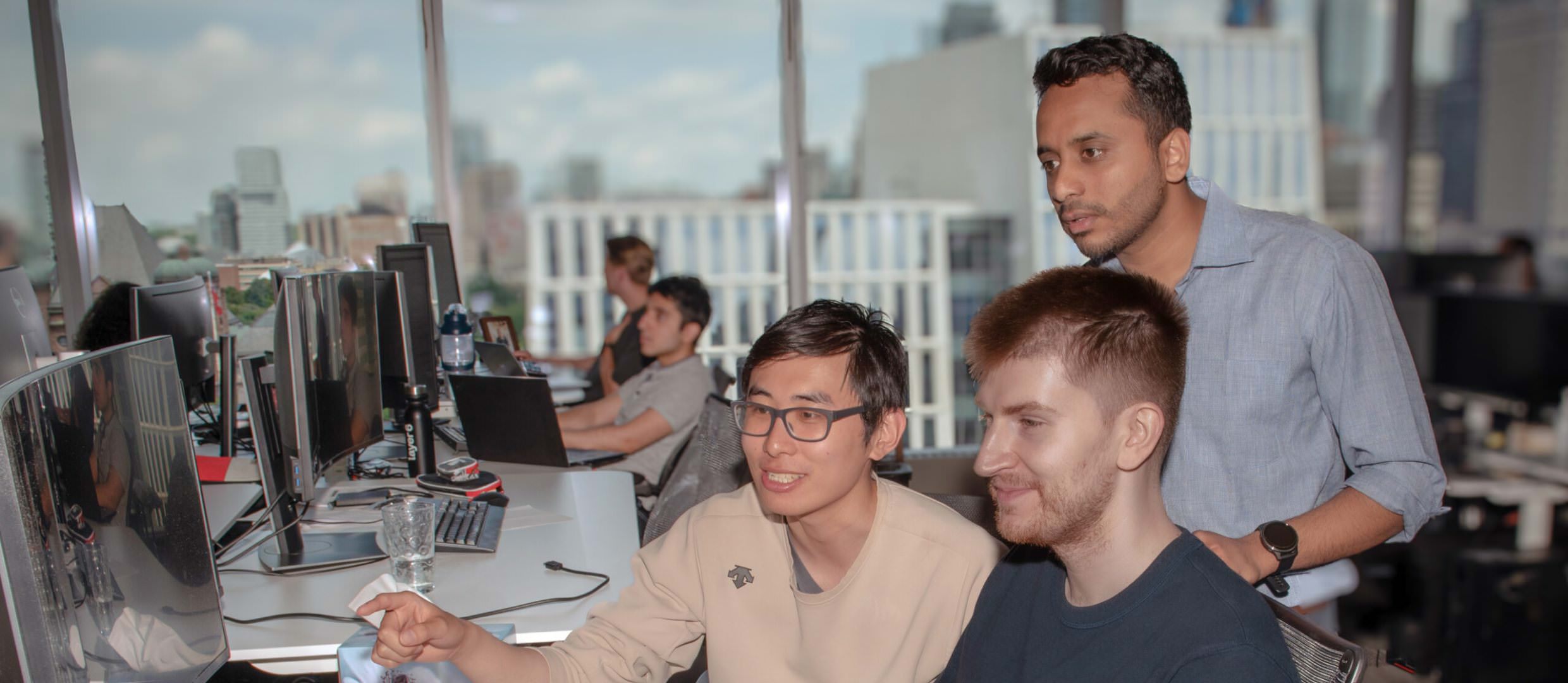
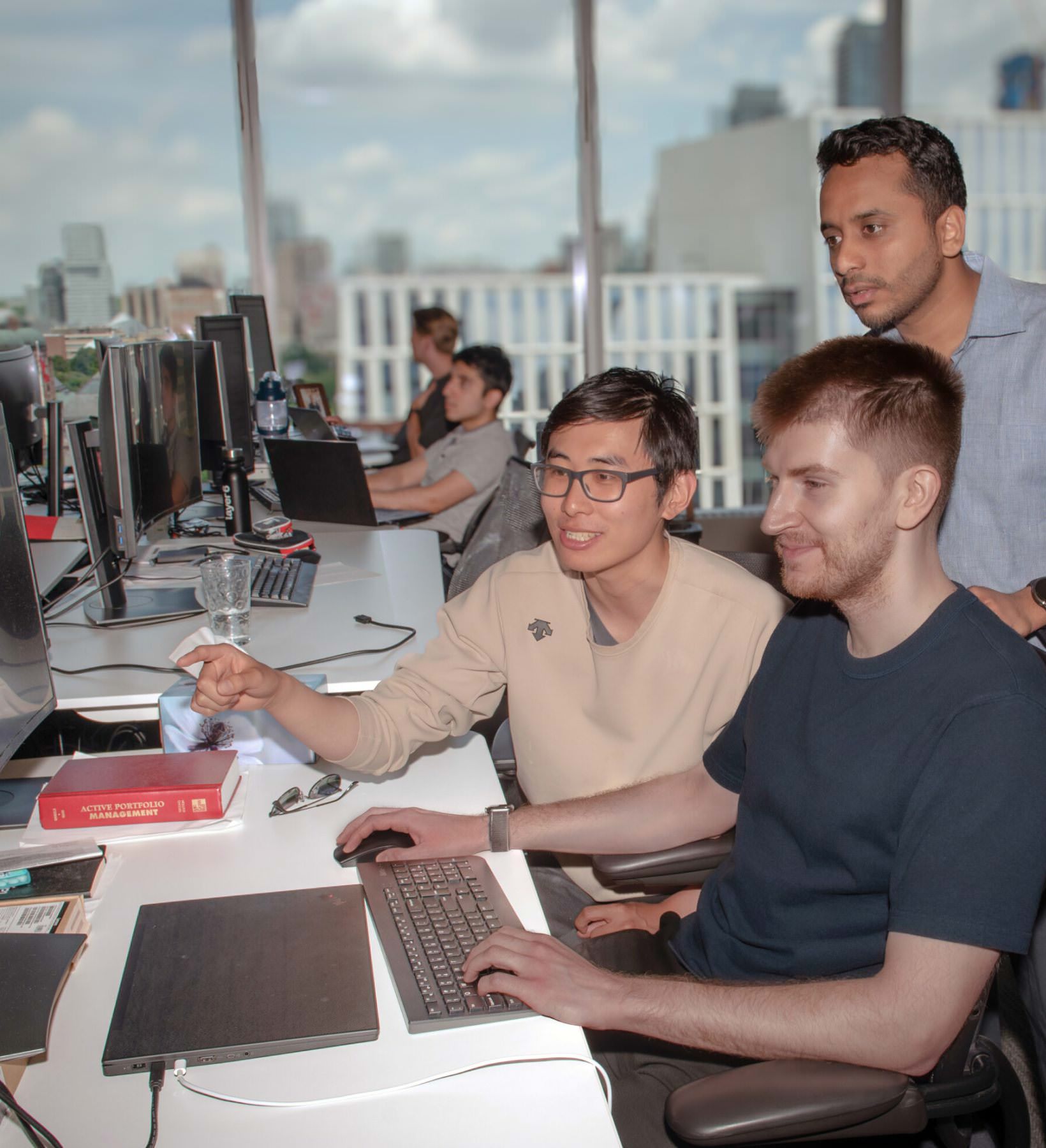
Ambitious applied research, positive outcomes
Layer 6 unites research, engineering, and product teams to quickly translate theory into impactful real-world applications.
Research Highlights
Our research is supported by access to massive datasets, close collaboration with world renowned academic faculty, and is deployed in impactful applications.
Our research areas include:
- deep learning and generative AI
- model explainability and trustworthy AI
- time series modelling
- natural language processing
Neural Information Processing Systems
NeurIPS 2024 Spotlight | A Geometric View of Data Complexity: Efficient Local Intrinsic Dimension Estimation with Diffusion Models
Abstract
High-dimensional data commonly lies on low-dimensional submanifolds, and estimating the local intrinsic dimension (LID) of a datum — i.e. the dimension of the submanifold it belongs to — is a longstanding problem. LID can be understood as the number of local factors of variation: the more factors of variation a datum has, the more complex it tends to be. Estimating this quantity has proven useful in contexts ranging from generalization in neural networks to detection of out-of-distribution data, adversarial examples, and AI-generated text. The recent successes of deep generative models present an opportunity to leverage them for LID estimation, but current methods based on generative models produce inaccurate estimates, require more than a single pre-trained model, are computationally intensive, or do not exploit the best available deep generative models, i.e. diffusion models (DMs). In this work, we show that the Fokker-Planck equation associated with a DM can provide a LID estimator which addresses all the aforementioned deficiencies. Our estimator, called FLIPD, is compatible with all popular DMs, and outperforms existing baselines on LID estimation benchmarks. We also apply FLIPD on natural images where the true LID is unknown. Compared to competing estimators, FLIPD exhibits a higher correlation with non-LID measures of complexity, better matches a qualitative assessment of complexity, and is the only estimator to remain tractable with high-resolution images at the scale of Stable Diffusion.
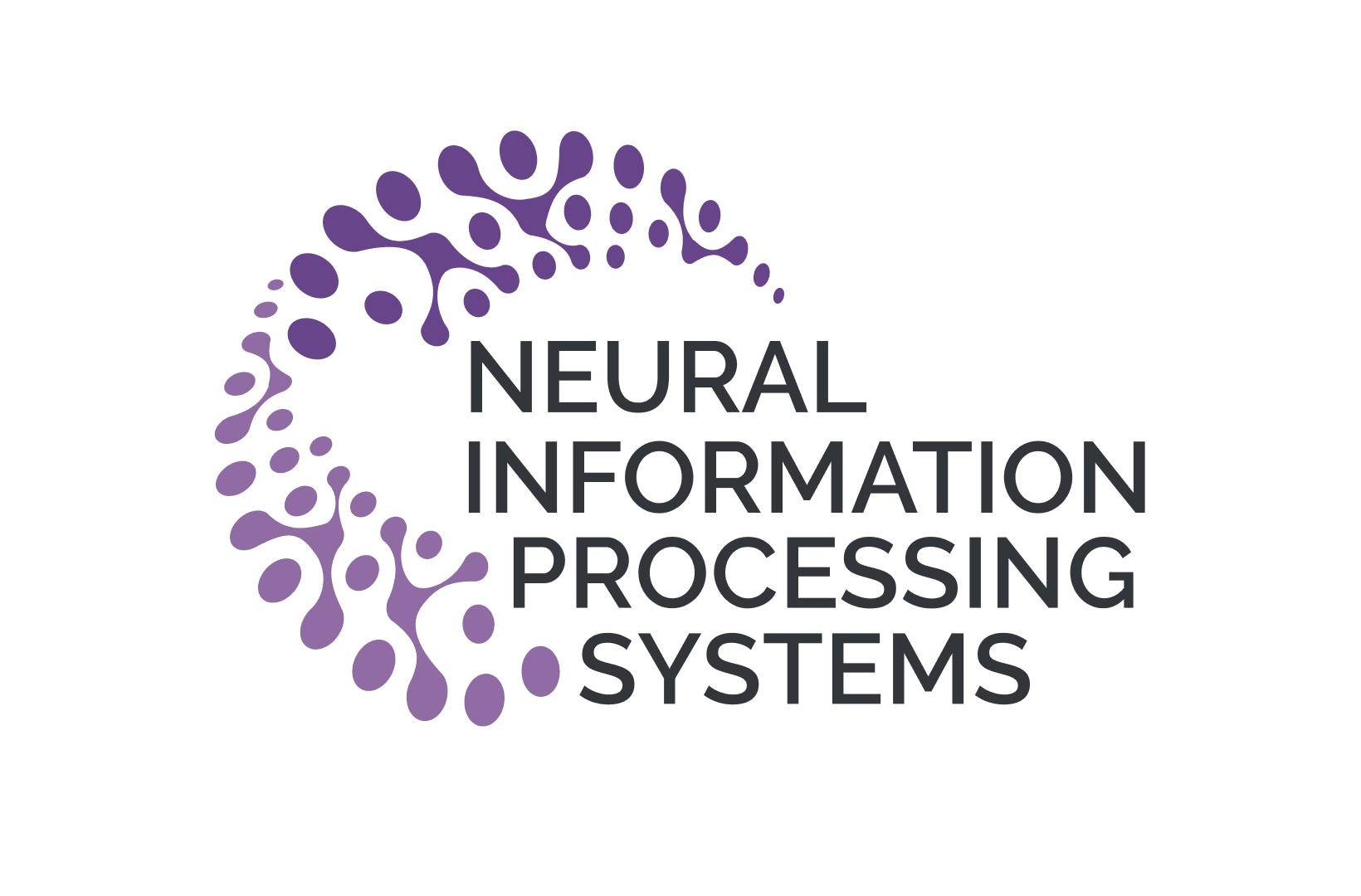
Neural Information Processing Systems
NeurIPS 2024 | Retrieval & Fine-Tuning for In-Context Tabular Models
Abstract
Tabular data is a pervasive modality spanning a wide range of domains, and the inherent diversity poses a considerable challenge for deep learning. Recent advancements using transformer-based in-context learning have shown promise on smaller and less complex datasets, but have struggled to scale to larger and more complex ones. To address this limitation, we propose a combination of retrieval and fine-tuning: we can adapt the transformer to a local subset of the data by collecting nearest neighbours, and then perform task-specific fine-tuning with this retrieved set of neighbours in context. Using TabPFN as the base model — currently the best tabular in-context learner — and applying our retrieval and fine-tuning scheme on top results in what we call a locally-calibrated PFN, or LoCalPFN. We conduct extensive evaluation on 95 datasets curated by TabZilla from OpenML, upon which we establish a new state-of-the-art with LoCalPFN — even with respect to tuned tree-based models. Notably, we show a significant boost in performance compared to the base in-context model, demonstrating the efficacy of our approach and advancing the frontier of deep learning in tabular data.
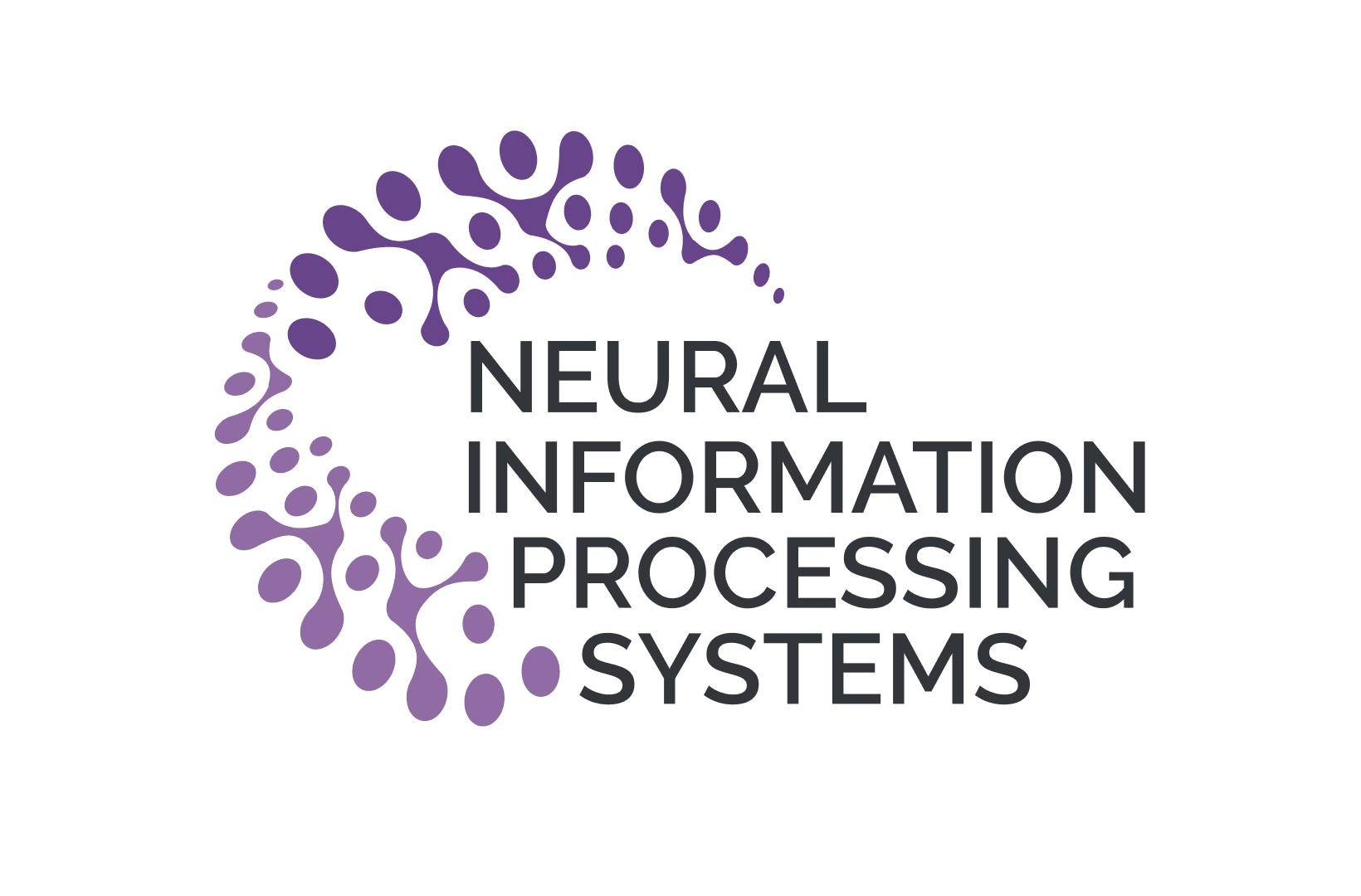
Transactions on Machine Learning Research
TMLR 2024 | Deep Generative Models through the Lens of the Manifold Hypothesis: A Survey and New Connections
Abstract
In recent years there has been increased interest in understanding the interplay between deep generative models (DGMs) and the manifold hypothesis. Research in this area focuses on understanding the reasons why commonly-used DGMs succeed or fail at learning distributions supported on unknown low-dimensional manifolds, as well as developing new models explicitly designed to account for manifold-supported data. This manifold lens provides both clarity as to why some DGMs (e.g. diffusion models and some generative adversarial networks) empirically surpass others (e.g. likelihood-based models such as variational autoencoders, normalizing flows, or energy-based models) at sample generation, and guidance for devising more performant DGMs. We carry out the first survey of DGMs viewed through this lens, making two novel contributions along the way. First, we formally establish that numerical instability of likelihoods in high ambient dimensions is unavoidable when modelling data with low intrinsic dimension. We then show that DGMs on learned representations of autoencoders can be interpreted as approximately minimizing Wasserstein distance: this result, which applies to latent diffusion models, helps justify their outstanding empirical results. The manifold lens provides a rich perspective from which to understand DGMs, which we aim to make more accessible and widespread.
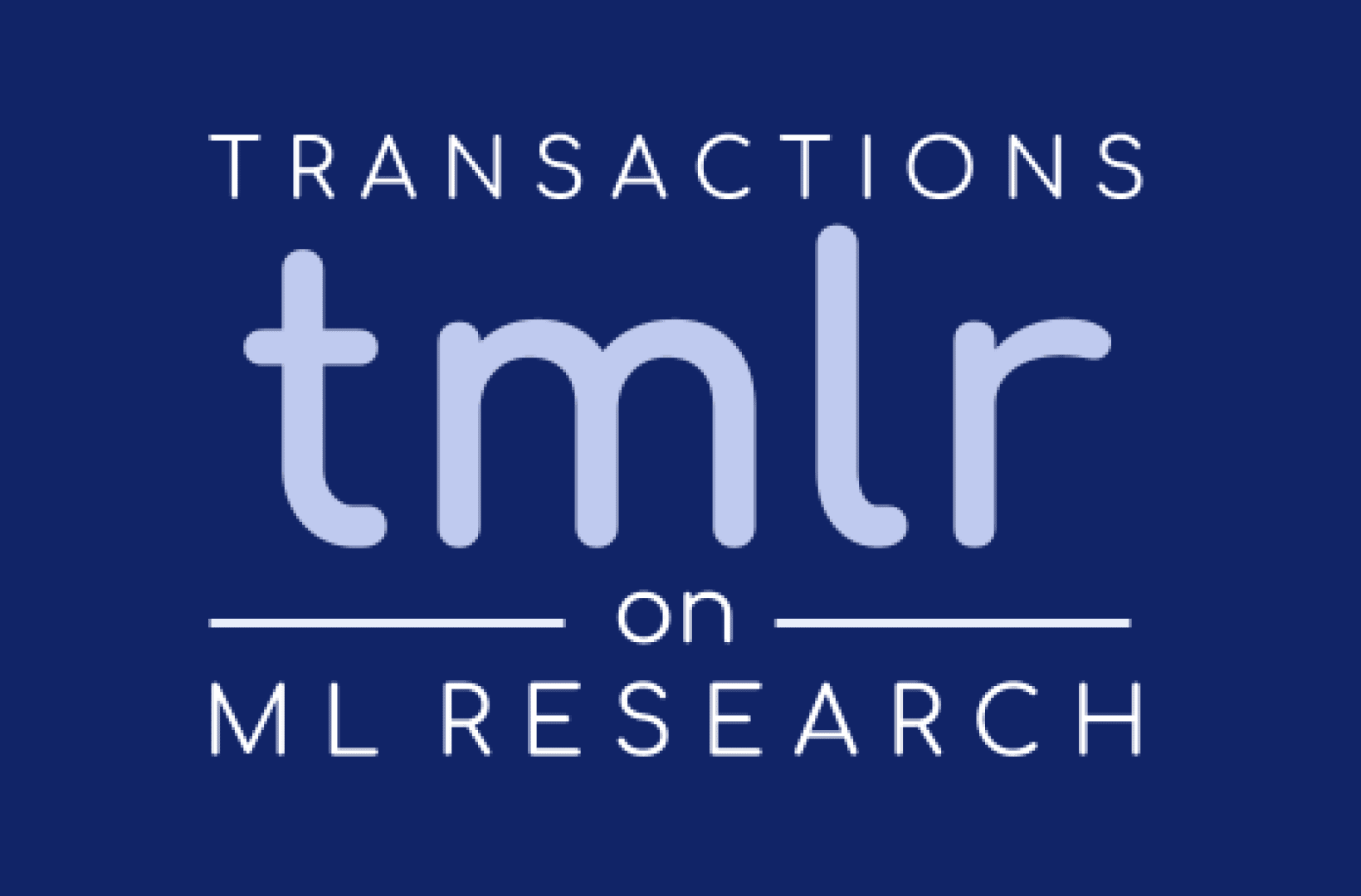
Transactions on Machine Learning Research
TMLR 2024| Augment then Smooth: Reconciling Differential Privacy with Certified Robustness
Abstract
Machine learning models are susceptible to a variety of attacks that can erode trust, including attacks against the privacy of training data, and adversarial examples that jeopardize model accuracy. Differential privacy and certified robustness are effective frameworks for combating these two threats respectively, as they each provide future-proof guarantees. However, we show that standard differentially private model training is insufficient for providing strong certified robustness guarantees. Indeed, combining differential privacy and certified robustness in a single system is non-trivial, leading previous works to introduce complex training schemes that lack flexibility. In this work, we present DP-CERT, a simple and effective method that achieves both privacy and robustness guarantees simultaneously by integrating randomized smoothing into standard differentially private model training. Compared to the leading prior work, DP-CERT gives up to a 2.5× increase in certified accuracy for the same differential privacy guarantee on CIFAR10. Through in-depth per-sample metric analysis, we find that larger certifiable radii correlate with smaller local Lipschitz constants, and show that DP-CERT effectively reduces Lipschitz constants compared to other differentially private training methods.
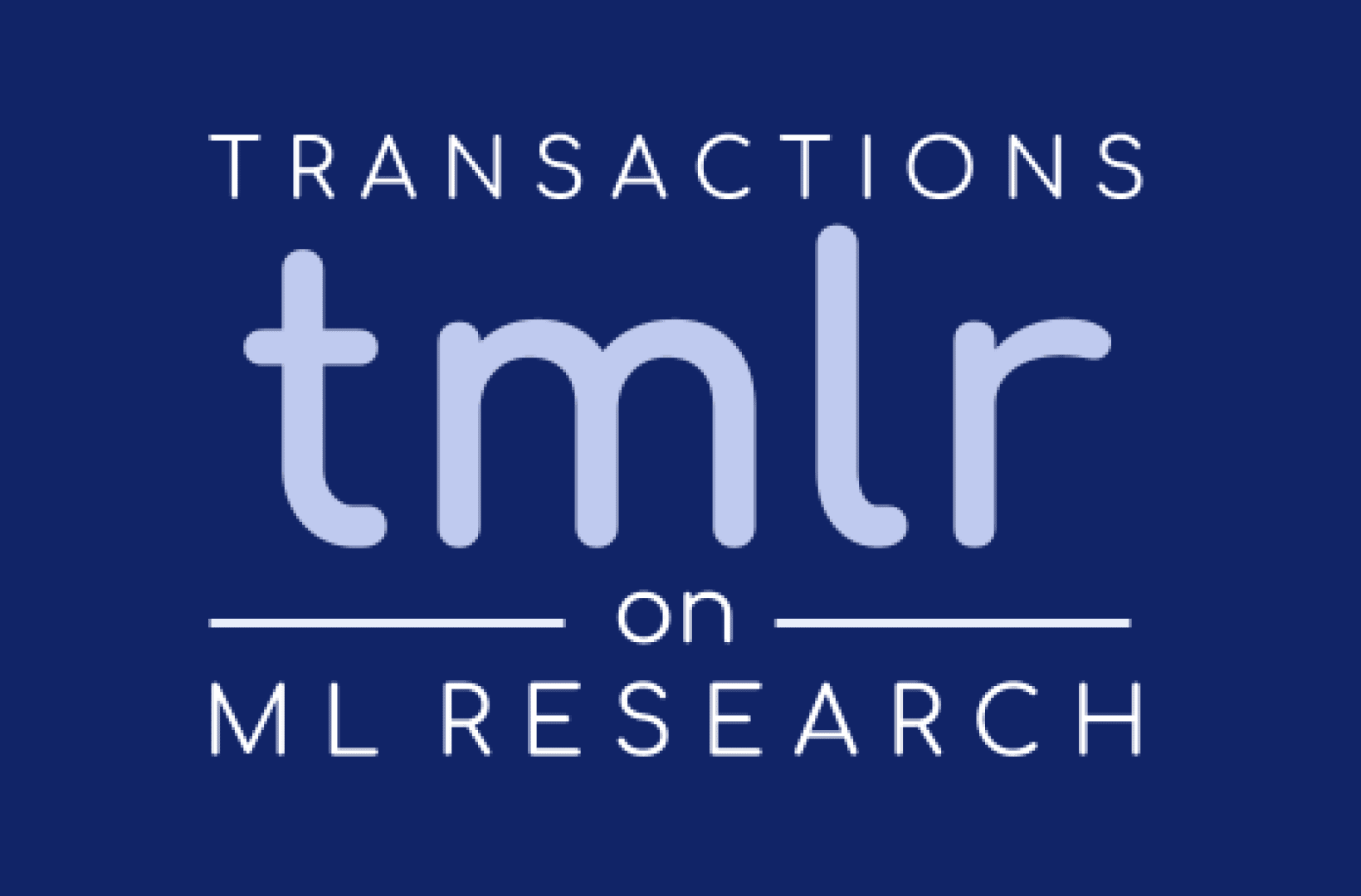
Computer Vision and Pattern Recognition Conference
DataCV Challenge 2nd Place | Classifier Guided Cluster Density Reduction for Dataset Selection
Abstract
We address the challenge of selecting an optimal dataset from a source pool with annotations to enhance performance on a target dataset derived from a different source. This is important in scenarios where it is hard to afford on-the-fly dataset annotation and is also the theme of the second Visual Data Understanding (VDU) Challenge. Our solution the Classifier Guided Cluster Density Reduction (CCDR) framework operates in two stages. Initially we employ a filtering technique to identify images that align with the target dataset’s distribution. Subsequently we implement a graph-based cluster density reduction method steered by a classifier that approximates the distance between the target distribution and source distribution. This classifier is trained to distinguish between images that resemble the target dataset and those that do not facilitating the pruning process shown in Figure 1. Our approach maintains a balance between selecting pertinent images that match the target distribution and eliminating redundant ones that do not contribute to the enhancement of the detection model. We demonstrate the superiority of our method over various baselines in object detection tasks particularly in optimizing the training set distribution on the region100 dataset.
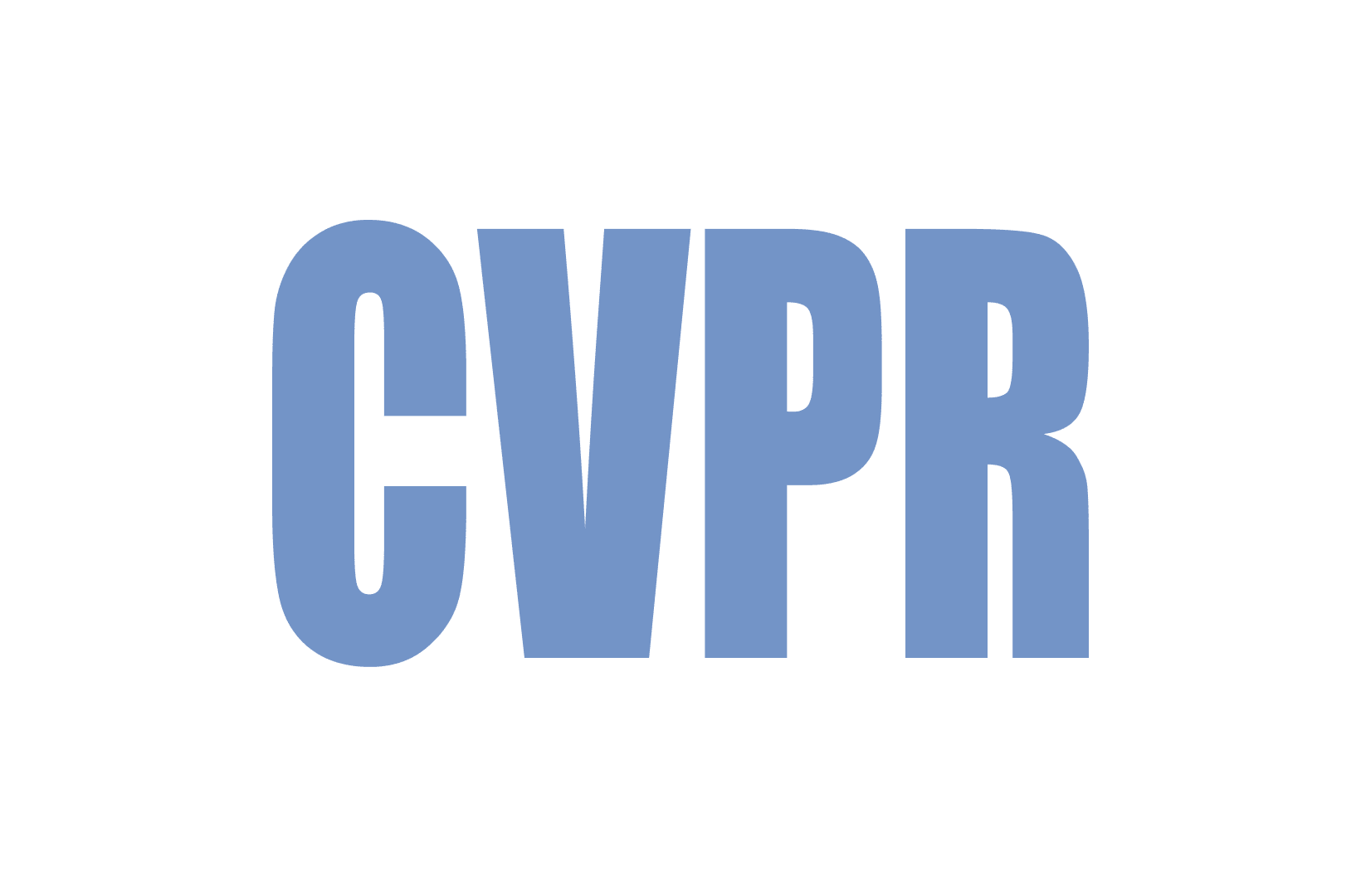
Our research areas include:
- deep learning and generative AI
- model explainability and trustworthy AI
- time series modelling
- natural language processing
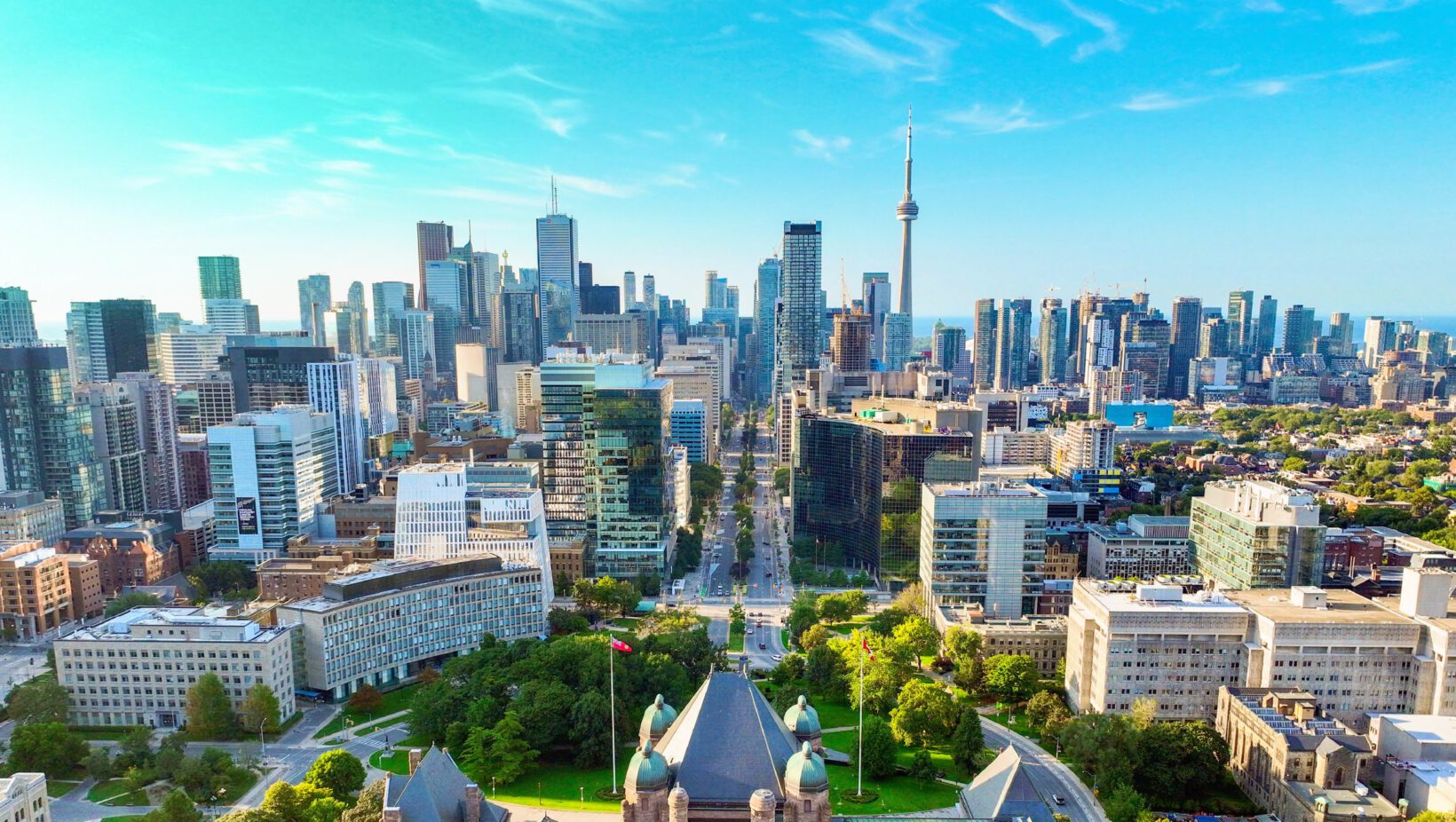
Big vision, deep roots
-
The founders of Layer 6 are also the founders of the Vector Institute for Artificial Intelligence, and we maintain active research collaborations with Vector faculty. -
Signal 1 spun out of the research collaboration between Layer 6 and St. Michael’s Hospital, and provides cutting-edge AI platforms for real time monitoring of patient outcomes. -
Radical Ventures was launched by the founders of Layer 6 to incubate and support leading AI startups in Canada and abroad. With over a billion dollars raised, Radical has become one of the premier AI focused venture funds in the world.
Impactful partnerships
Originally founded in 2011, Layer 6 now forms the AI centre of excellence of TD Bank Group. Layer 6 impacts the lives of over 27 million customers, helping more people achieve their financial goals and needs through AI systems founded on the responsible use of AI.
Layer 6 embraces opportunities to contribute to the Canadian AI ecosystem. The founders of Layer 6 played pivotal roles in launching the Vector Institute, Radical Ventures, and Signal 1. Together these entities are integral in driving the Canadian AI innovation, from research to product incubation to scale-up. We continue to collaborate with leading academic institutions globally.
Passion to learn, driven to succeed
Our team comes from globally diverse backgrounds and we care deeply about fostering an inclusive culture. We learn from each other and win together. We are united by our passion for deep learning and a desire to apply our skills to have an outsized and positive impact on the future.
Meet some of our team
Develop your career at Layer 6
We’re growing our team with people driven to be at the cutting edge of machine learning in research, engineering, and impactful applications.
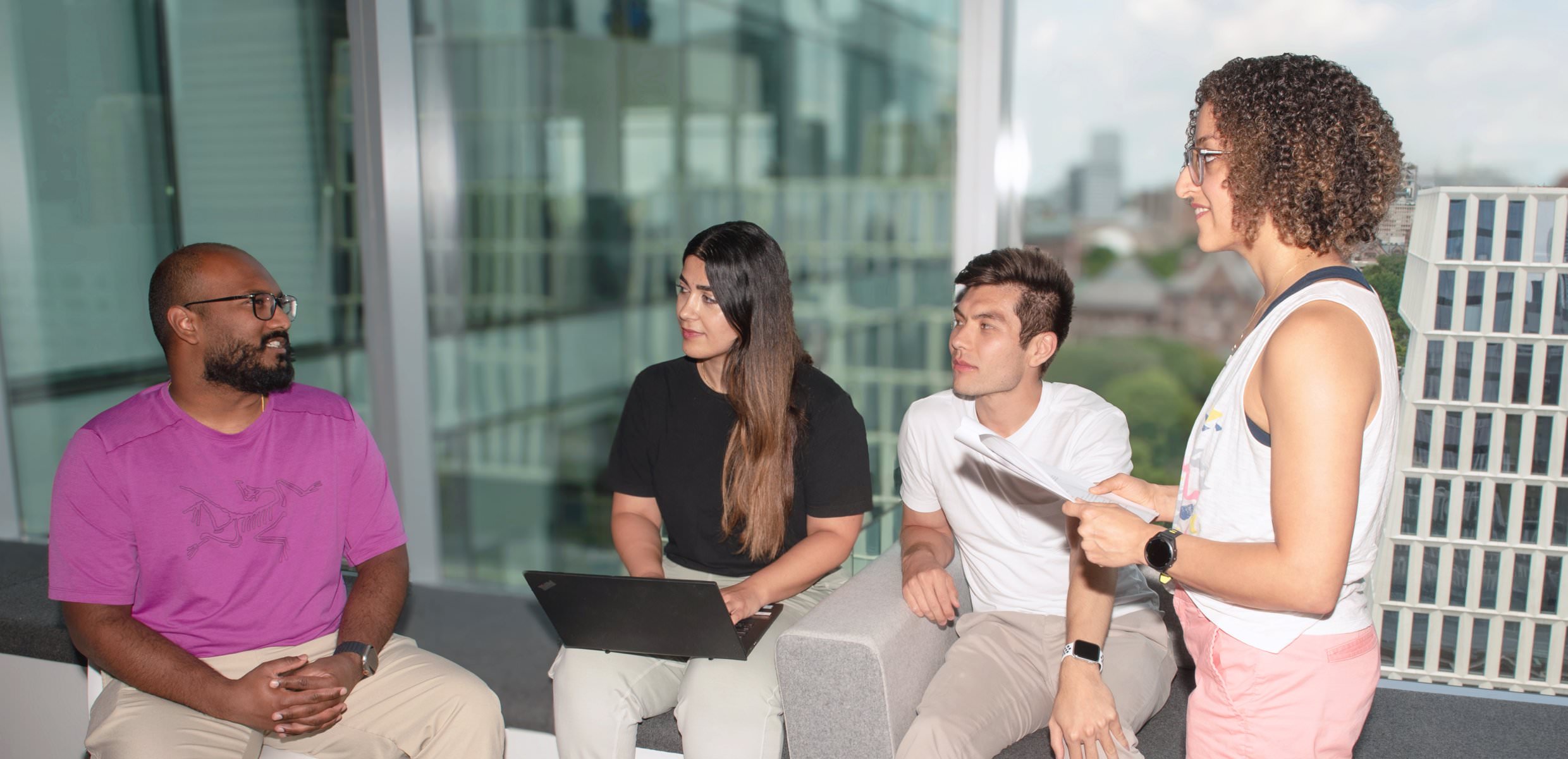